A Deep Look into The Power of Analyzing Sources of Error in The Lab
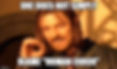
A Missed Opportunity
As a high school science student, I was required to write up labs and this was usually done on some type of handout. There was often a question at the end that asked me to think about potential sources of error. I would jot down things like “air drag,” “friction,” or (dear God, this is the worst one) “human error” and that was enough to get the points. My teachers didn't take time to really read what I'd written, nor did they discuss these potential sources of error and what their implications might be in relation to the claim I was trying to make. My teachers were focused only on whether I understood the relevant scientific model and how it applied to the experimental system and made little effort to encourage us to think deeply about the model's shortcomings.
Teaching students to evaluate the validity of a physical model within the context of an experiment is a crucial science practice. As a prerequisite, this process requires students to understand the relevant model and the experimental system. It also requires them to use common sense, evaluate the significance of factors unaccounted for in the model, and to scrutinize their data.
Students should be digging into their data to uncover how, and to what degree, a model fails—because to some extent it always does.
Error Analysis Through Quantitative Comparison
I don't ask students to reflect on errors for every lab because doing it properly is time-consuming and requires significant effort. When I do, I expect them to make strong claims about sources of error utilizing logical reasoning and their data as evidence. This is achieved by requiring students to make quantitative comparisons regarding their experimental outcomes.
Take for instance a lesson where students perform an experiment and analyze their data to calculate an unknown physical constant. Students could then be asked to make a claim about whether their experimental value is likely to be greater or less than the actual value and to support their claim with logical reasoning evidenced by their data.
The quantitative element is essential because it requires students to think carefully about relevant equations and how a particular source of error will "propagate" through them. Students need to think about which variables are "inflated" or "reduced" as a result of unaccounted for, real-life factors or measurement inaccuracies. I do NOT require students to calculate margins of error. This process is too time-intensive, diverges too far from the AP curriculum, and simply doesn't require a much deeper understanding than having students do just a quantitative comparison.
Note that this can also be achieved in experiments that are more inquiry-focused although it looks a bit different. Consider a situation where students conduct a controlled experiment in a system for which a mathematical model has not yet been developed. Let's say their data yields a line. Even though they haven't yet learned the model that would equip them to explain why it's a line, they can still think carefully about the system and make claims about whether the intercept and slope are greater or less than they "should" be. This can be done by examining factors they tried to minimize/hold constant (but weren't) or experimental errors in measurements that were taken.
Expectations, Assessment, and Guidance
I ask students to keep lab notebooks. I give them general guidelines for each lab, but I allow them to organize their work in whatever manner suits them so long as they meet expectations. When assessing their error analyses, I actually use a rubric designed specifically for this purpose (scroll down to LS4).
In their error analysis I expect students to do the following:
Discuss at least one significant source of error and connect it accurately to experimental outcomes through use of quantitative comparisons.
Propose sources of error that are supported by logical reasoning and their data. These sources of error should also be feasible in the sense that they are likely to have actually had a significant effect. (This could be just a single source of error as long is it is the most significant and there are no other major ones.)
If the data set has insufficient range, notable outliers, or low precision/accuracy, this is mentioned and possible reasons are discussed.
It can be hard teaching students to think carefully about their data. Often, because there is often no single right answer and it makes them uncomfortable when there's no "right" way to do it. Data may be different from one group to another and this means that students need to think carefully about their own results. They can't simply borrow ideas from their friends in the class.
Early in the year I introduce the Spherical Cow to illustrate how physical models do not perfectly represent reality. I also give them this document (How to Write an Awesome In-Depth Error Analysis) to reference throughout the year if they don't know what to write about. Students often get stuck looking at their data in one way. This guide is designed to give students a few different look-fors to help them critique their data from multiple perspectives in the hope of determining the most relevant approach to take in writing about potential errors.
I also try to build in some time for students to discuss potential sources of error at the end of lab day. I do my best to refrain from discussing them myself, because I want the students to uncover them on their own, but sometimes I will push them in the right direction if there is a distinct error source that I feel is too important for them to miss.
Still, students get stuck and come in during lunch stumped on what to look at. I find myself repeating statements like the following:
Look at your graph! Is it what you expect?
Your graph has a vertical intercept. Does it make sense for there to be an intercept?
Do you think this source of error would cause your graph to be shifted or stretched? Does it affect the intercept or the slope?
Sure, there was probably some air drag, but based on your data is it substantial enough to affect your results?
Think about the way you collected your data--are you likely to have consistently measured this incorrectly? Could this be caused by some type of systematic error?
Was the sensor zeroed out properly? Maybe this relates to what you're seeing.
Student Samples
I have tried to include examples from a diverse set of experiments to provide an idea for what this looks like in different contexts.
Student Sample 1: From Resistivity of Clay Lab (AP Physics 1)
Below is an example from the resistivity of clay lab that I wrote about in another post. I would consider this to be pretty close to the gold standard although there is still some room for improvement.
The student goes above and beyond and identifies three sources of error and connects them to experimental outcomes. Although this student could have improved their work by making connections more explicit.
The student first identifies that the clay is drying out and that over time this would have caused the resistivity to increase. The student uses common sense to make this claim, but also relies on data to provide evidence as support.
Next, the student talks about the nails in the clay and explains that this affected the "route" of current flow through the clay. Since the current enters and exits at the nails, current flow throughout the clay will not be uniform. The student argues that this will change the effective cross-sectional area, essentially making this constant variable less than what it was measured to be. I would like to see this student go a step further and argue whether this source of error would have caused his calculated resistivity value to come out greater or less than the actual value.
The student also makes a claim about the resistance of other parts of the circuit. The student is right that this unaccounted-for resistance would cause the experimental value for resistivity to come out greater than the actual value, yet this is not articulated in a particularly clear manner. It's also worth questioning whether the resistance of the rest of the circuit is high enough to be significant enough to noticeably affect the experimental results.
Sample 2: Rotational Inertia of a T-Pipe (AP Physics 1)
In this experiment, students had a T-shaped set of PVC pipes. Students were required to find the inertia of the T-pipe in two ways:
Direct Measurement Method: by breaking it apart and measuring the mass and relevant dimensions of each piece. Then they used a rotational inertia table to calculate the inertia of each component. Finally they had to sum the pieces to find the inertia of the whole object.
Experimental Method: They also had to conduct a controlled experiment in which they measured its resistance to angular acceleration. They were then required to create a graph of their variables and analyze their graph in a manner that would allow them to calculate the rotational inertia of the pipe.
For their error analysis students were required to compare their direct measurement measurement value and their experimental value and discuss reasons for the discrepancy. Here's a link to the student task sheet if you want to take a look.
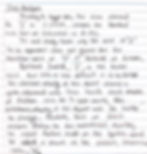
This student provides a reason for the discrepancy between the two values (friction) and does a relativity good job of communicating why friction likely caused the experimental value to come out greater than the direct measurement value. This is a simple, yet strong response because the student uses logical reasoning and experimental evidence (the two rotational inertia values) to support their claim. Note that the student writes "theoretical value" for what I've referred to as "direct measurement" value.
Sample 3: Finding Focal Length (AP Physics 2)
In this lab I gave students a converging lens and a diverging lens with the task of experimentally determining the focal length of each lens by conducting controlled experiments and creating graphs. Students had access to a candle and an optics bench on which the could measure object and image distance by focusing candlelight on a card. This is a bit tricky for the diverging lens because on its own, it doesn't produce a real image; instead you need to set up a two-lens system for this portion of the experiment. See the student task sheet here (it's black because this is performed in the dark).
For the error analysis portion, students were given the actual focal lengths of the lenses and asked to compare these with their experimental values.

This is an outstanding example. The student points to a specific outlier and addresses it by trying the analysis with and without it to see how much its presence affected the experimentally determined value for focal length. The student also provides a reason for why the data points lacked precision (writing "skewed to either side") by explaining that it was difficult to tell exactly where the image was most in focus.
The student points out that the experimentally determined focal length for the diverging lens was farther from the actual value than the focal length of the converging lens and proposes a feasible reason for why this occurred. The student explains a systematic source of error and how it affected the graph and the subsequent calculations. This is exactly the type of "quantitative comparison" that I hope to see.
This student even goes to the trouble of playing with the numbers a little and seeing how much a 1mm error would affect the final result in order to further justify that these small measurement imprecisions would cause significant effects.